Why "generic GPT" can't write your next email
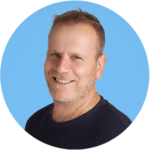
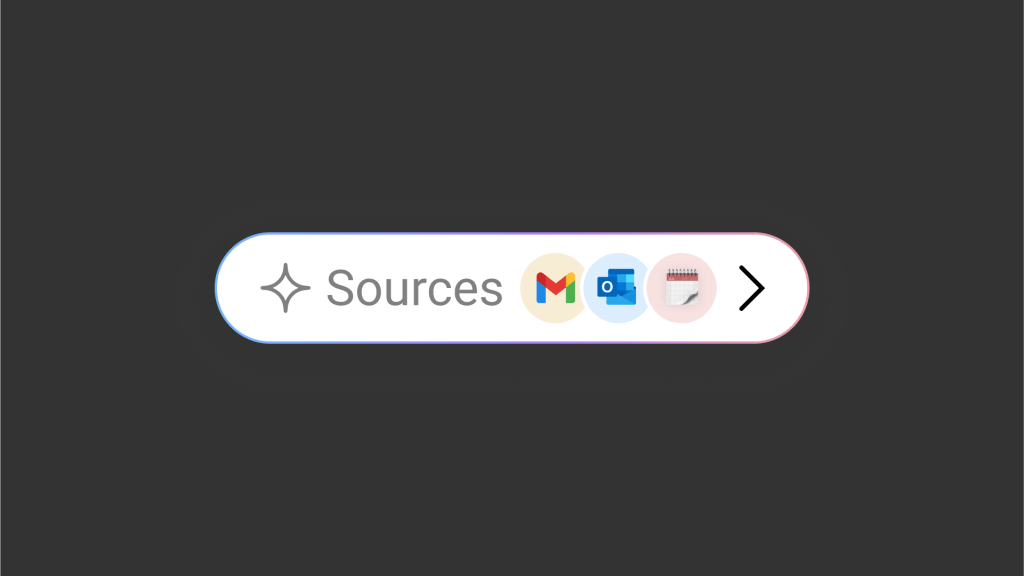
So you can use AI software to write all your emails, or so it seems. The premise is simple: copy-paste the email you got, add “write a reply,” and the GPT spits out a passable response with plenty of em-dashes, sharp transitions, and scattered phrasing.
If you want to go the extra mile, you even provide some context. “Write me a reply that gives them a distribution plan for the materials they need,” or whatever. Then you get a reply with the context you asked for, still plenty of em-dashes, and a [hey name] that you may embarrassingly forget to remove.
Yes, you could provide this context in the prompt, but is that more worthwhile than just writing an email? If not, then what’s the point? Just do it yourself, it’ll almost certainly be better written and take the same amount of time.
This isn’t efficiency, it’s just outsourcing the first draft to a system that has no idea what you’re talking about. The time you spend correcting AI-generated content often exceeds what you’d spend just writing from scratch with full knowledge of the context. Instead of delegating the task, all you’re really doing is outsourcing the guesswork.
This leaves you with the burden of extensive editing and refinement. And so, instead of enhancing productivity, they introduce a new form of labor, editing the AI output, which is in itself a time-consuming task.
What if there were a better way? What if you could have AI write all your emails, and they’re actually good? This may be possible, but first, let’s understand what’s causing the issue.
Why contextual AI fixes the problem
Generic LLMs, despite their impressive capabilities, are trained on everything except you and your specific circumstances. Without access to your personal and business context, these systems don’t genuinely compose, they merely generate plausible text based on patterns that they’ve observed elsewhere.
These models have no access to your most important information sources, such as your email history, documents, CRM data, and customer interactions. They also don’t know your brand’s unique voice, tone, and mission.
But what if the AI actually had access to the information it needs?
Contextual AI approaches this differently by pulling in your real-time data: your past emails, documents, meeting notes, CRM records, and project histories. Instead of guessing based on internet patterns, it adapts to your voice, workflows, and specific situation.
It doesn’t just generate text; it retrieves relevant information first and then composes something that actually makes sense for your specific situation.
The difference becomes obvious when you compare outputs:
Prompt: “Write a follow-up to last week’s pricing discussion with Jane.”
Generic GPT:
“Hi Jane, I wanted to follow up on our previous conversation about pricing. I’ve given some thought to the points you raised, and I’m interested in continuing our discussion. Please let me know when would be a good time to connect.”
It’s grammatically correct, polite, but utterly devoid of value. It could be about any product, pricing tier, objection, or relationship. Jane could be a prospect or a vendor. The AI has no idea, so it hedges with vague language that you’ll have to completely overhaul anyway.
Contextual AI:
“Hi Jane, Following up on our discussion about the Enterprise tier pricing for TeamFlow last Thursday. I’ve prepared the custom pricing model that addresses your concerns about the per-seat cost at your scale. As you requested, I’ve included the volume discount structure starting at 250 users. Would you have time this Friday at 2pm to review these updates, as you mentioned that’s your preferred meeting time?”
Notice the difference?
How it works: Retrieval before generation
The technology behind this isn’t magic. At its core is a process called Retrieval-Augmented Generation (RAG), which works like having an assistant who checks your files before writing anything.
First, the system creates “embeddings” from your documents and emails like digital fingerprints that capture the meaning of content, not just the exact words. This means the system can find information about “pricing concerns” even if the original document used terms like “cost issues” or “budget constraints.”
Then, when you ask it to draft something, it searches through these fingerprints to find relevant documents, extracts the important details, and only then generates a response that incorporates this specific information.
Privacy controls ensure information stays within appropriate boundaries, so your data stays private while becoming actionable.
It doesn’t try to sound right. It tries to be right.
What are the real-world benefits?
Contextual AI delivers meaningful improvements across several key areas:
For sales teams:
- Reduce time spent drafting follow-up emails with accurate responses that reference specific customer pain points and objections.
- Improve the quality of proposals by automatically incorporating relevant case studies and specific pricing models.
- Ensure consistent messaging across the entire team while maintaining individual relationship contexts.
- Spend less time searching for information and more time building customer relationships
For customer support:
- Decrease resolution time by having immediate access to all previous customer interactions.
- Improve response consistency with automatic inclusion of accurate product information and company policies.
- Reduce escalations through more complete initial responses.
- Help new support staff perform like veterans from day one.
For knowledge workers:
- Eliminate hours spent searching for information across siloed systems.
- Reduce time spent preparing for meetings with better communications that incorporate all relevant context.
- Improve onboarding efficiency by giving new employees immediate access to institutional knowledge.
- Focus mental energy on high-value thinking instead of routine composition.
Use cases across levels
So what does this look like in practice?
Imagine an AI that writes emails that actually sound like you because it’s learned your writing style from your sent folder. It summarizes long threads accurately because it can actually read them. Most impressively, it might say something like “Remember to include the updated pricing sheet you promised Jane last week” because it knows your commitments.
The benefits multiply when your whole team uses contextual AI. New team members get up to speed faster because the AI helps them access institutional knowledge. Customer support becomes more consistent because everyone has access to complete customer histories.
Sales proposals reflect your actual positioning and differentiators, not generic sales language. A major advantage is searchable decision memory, being able to quickly answer “Why did we decide on this policy last year?” without digging through endless meeting notes or depending on whoever has been at the company longest.
Larger organizations face even bigger challenges with information silos. Contextual AI can provide compliant, secure, role-based responses where access is governed by existing permission structures. The system never sees what it shouldn’t see, preserving necessary information barriers while making institutional knowledge searchable and useful.
For enterprise buyers, this means zero data leakage. Your sensitive information stays within the boundaries you’ve established, with RAG operating under scoped access controls—many leaders care less about the generative magic and more about proper containment of proprietary information.
The strategic shift: From output to understanding
The counterintuitive truth is that building bigger, more powerful language models won’t solve this problem. A model with twice as many parameters will just make more confident guesses, not more accurate ones.
Context multiplies value. Scale without context multiplies noise. The same model delivers dramatically better results when it has access to relevant information. This is the inflection point where AI shifts from “interesting toy” to “genuine productivity tool.”
The strategic implications for forward-thinking organizations are clear.
Companies focusing exclusively on model size or general capabilities are investing in the wrong metrics. The true competitive advantage comes from three key elements:
- Integration systems that connect general language ability with your specific organizational knowledge.
- Secure frameworks that maintain privacy while activating your proprietary information.
- Contextual awareness that allows AI to understand the full picture of your business relationships.
Without these elements, even the most sophisticated language models will continue to produce outputs that require substantial human correction, undermining their promised efficiency.
Conclusion: The future writes with you
You don’t need a more eloquent stranger writing your emails. You need a partner that actually understands your work, voice, and context.
This makes the difference between AI that creates additional work through necessary editing and AI that actually completes tasks effectively. It’s not just a technical distinction, it’s the difference between frustration and actual productivity.
AI is only as smart as what it knows, and right now, generic AI doesn’t know you or your business. That’s the fundamental limitation we need to overcome.
The next wave of real productivity won’t come from marginally better generic language models. It will come from contextual AI systems that know your work as well as you do, ground their outputs in your reality, and genuinely augment your capabilities rather than just approximating them.
Until it knows your world, AI isn’t your assistant. It’s a stranger with a keyboard.