Why most AI tools fail to solve real workplace problems (and how to fix it)
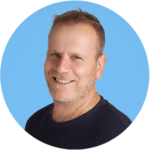
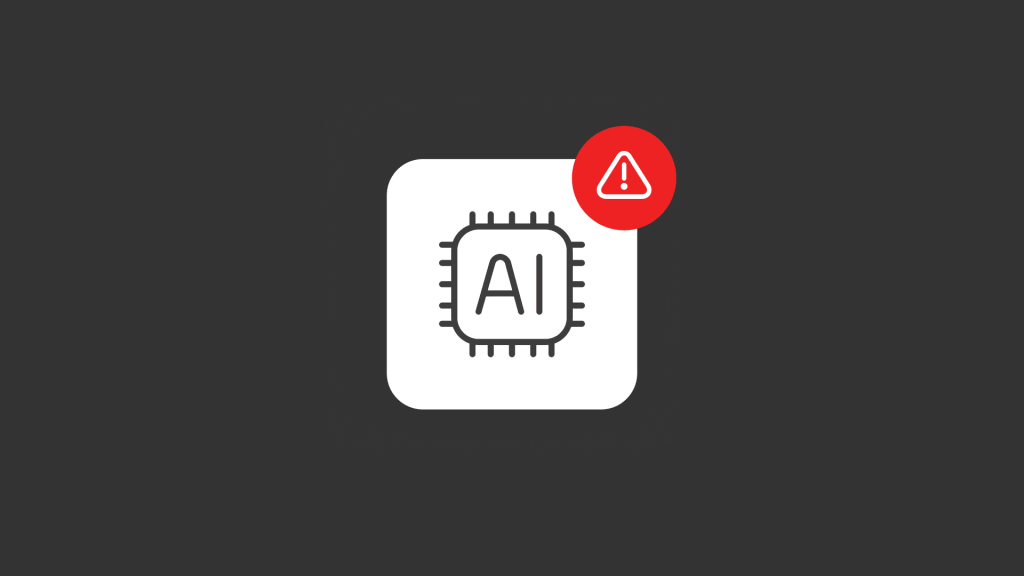
“I spent more time explaining what I needed than it would have taken to do it myself.”
This frustration has become the defining experience of AI in today’s workplace. What promises to save time often ends up consuming it instead. Professionals find themselves trapped in a cycle of detailed prompting, constant correction, and endless context-building, only to end up with outputs that still fall short.
The issue is AI’s blindness to context, specifically its inability to understand who you are, what you’re working on, and what decisions have already been made. Without this context, even the most powerful AI becomes another drain on time and focus rather than the productivity tool it was meant to be.
How the isolated AI interface creates chaos
The root of the problem is that AI is not integrated into our work. Most AI systems operate as standalone tools in what we call the search box vacuum, a disconnected interface where every interaction starts from scratch.
In this vacuum:
- You must repeatedly explain the basic context with each new prompt.
- Your previous conversations and preferences are no longer remembered.
- The AI exists entirely outside your actual workflow and tools.
- You’re forced to manually bridge the gap between AI outputs and real work.
AI today can analyze complex research papers, but can’t remember what you told it yesterday about your project timeline. It can write elegant prose, but can’t recall your company’s tone guidelines. It excels at general knowledge but fails at the specific details that make work meaningful.
This disconnect undermines the very efficiency AI promises. Each time you switch to an AI tool, you must translate your work context into explicit instructions, then translate the output back into your workflow.
Better prompts can’t fix a broken system
The default response, when facing AI-related issues, is “Let’s learn how to write better prompts.” Teams create elaborate prompt templates. Employees are encouraged to be more precise, more detailed, and more strategic in their AI interactions.
No matter how meticulously crafted, a prompt cannot make an AI know things it was never trained on. Your company’s meeting history, client relationships, project status, and strategic priorities are completely invisible to a model trained on general data.
Even with the “perfect prompt,” you can’t compress a complex web of company knowledge and project history into a text instruction. Lacking this background, the AI will inevitably miss crucial elements of what you meant.
The real-world consequences are painful:
- Spending 20 minutes crafting the perfect prompt only to get a generic response.
- Manually correcting the same company-specific facts in every AI output.
- Watching your supposed “time savings” evaporate as you endlessly revise AI-generated content.
Ultimately, the math simply doesn’t work: the time spent explaining, correcting, and revising often exceeds whatever time they saved by using AI in the first place.
The four elements of effective contextual AI integration
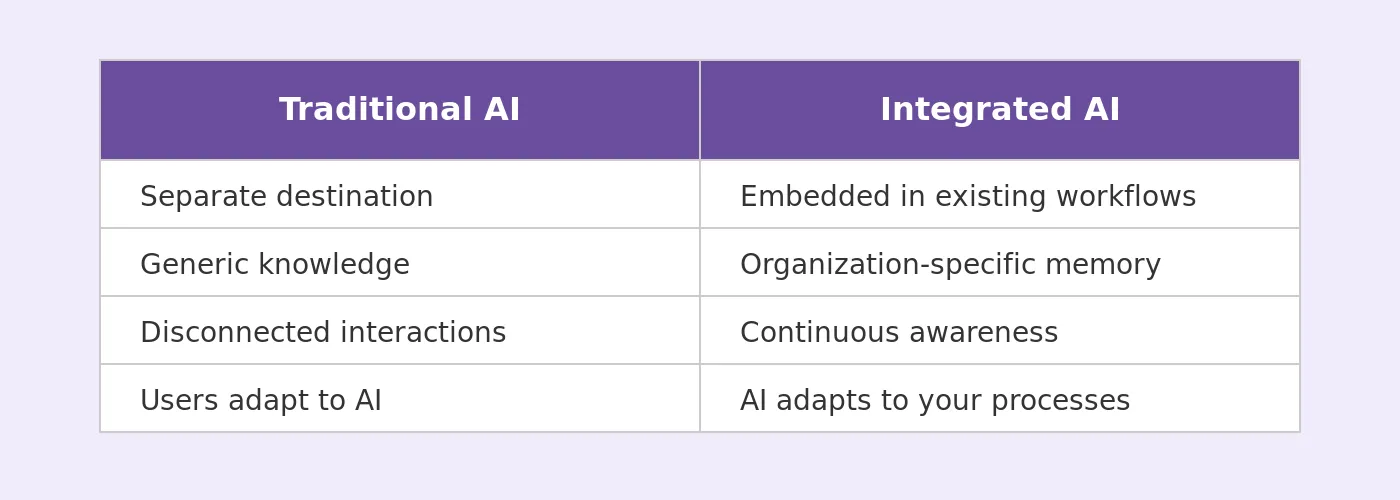
True workplace AI must address context across multiple dimensions simultaneously. Each component builds upon the others to create systems that understand your work as well as your human colleagues do.
1. Information that connects to your work
Scattered documents, emails, databases, and chat logs create chaos. For AI to work effectively, it needs to understand how information connects what drives decisions, which teams are responsible for specific tasks, and how today’s work builds upon the past. Without that map, AI treats every task as if it’s starting from scratch.
Building these connections isn’t easy. Many organizations face data silos and security hurdles that keep information locked away. Still, breaking down these barriers is the first step to making AI truly context-aware.
2. AI that lives inside your tools
When AI is built into your existing tools, you stay focused without constantly switching apps. Instead of interrupting your workflow, AI quietly supports it.
Picture writing an email as usual, and AI suggestions are uniquely tailored to your past conversations. You don’t have to think about using AI; it just works in the background, helping you stay in the flow.
3. AI that remembers your history
Human teams rely on history; today’s meeting builds on the decisions made last week. But most AI systems start from zero every time.
AI with persistent memory can track project histories, client relationships, and past decisions, growing its understanding over time. Without memory, users are stuck constantly re-explaining their work, especially on long projects where context keeps building.
While true memory in AI is still developing, real progress is being made. The goal is clear: systems that learn from past interactions and build a lasting understanding of your work.
4. AI that understands your team dynamics
For AI to truly fit into the workplace, it must understand communication styles, decision-making habits, and personal preferences. It needs to know that an email to the CEO sounds different from a team update, and that some people prefer charts while others want detailed reports.
Social awareness also means knowing your company’s culture and unwritten rules. Without it, AI may technically answer questions but miss the human side of work.
Companies that build all four layers of context information, process, memory, and social understanding, see significantly better results. Context isn’t optional; it’s what makes AI truly valuable.
The cost of AI context blindness
Missing context creates real business problems that get worse over time:
- Strategy disconnects: AI often produces outputs that miss company goals because it can’t see strategic plans. Teams then waste time realigning work.
- Worker frustration: After the initial excitement, employees become frustrated when AI tools fail to understand their projects and require constant corrections.
- Team misalignments: When teams utilize disconnected AI, they obtain inconsistent results, resulting in confusion and misaligned work.
- Poor decision quality: AI often suggests ideas that sound good in theory but fail in practice because it misses important past decisions, limits, and needs.
Making AI actually understand your business
Leading organizations are already shifting from “What can AI write for us?” to “How can AI improve our specific work processes?” This transformation focuses on systems that:
- Connect directly to your company’s knowledge, not just public data.
- Learn continuously from your organization’s patterns.
- Remember information across conversations and time.
- Adapt intelligently to your unique working style.
When done correctly, context-aware AI saves time, enhances work quality, and boosts employee satisfaction. Workers spend less time searching for information because the system already knows what matters and produces better results by following company-specific guidelines and history.
The AI context revolution
The future belongs to organizations that understand a key truth: intelligence without context isn’t real intelligence. It’s just raw calculation, impressive, but not truly useful.
Bridging the gap between AI’s power and human understanding takes careful planning. It’s not easy, but it’s necessary for those who want real results.
While we’re developing comprehensive solutions addressing these issues, here are three immediate actions you can take:
- Review information flows: Map where key knowledge resides, how it is shared between teams, and identify any existing gaps.
- Choose integrated tools: Select AI that seamlessly integrates with your current systems, even if it means fewer features at first; preserving context is more important.
- Establish information-sharing standards: Implement simple habits for documenting decisions and capturing knowledge to lay a stronger foundation for future AI systems.